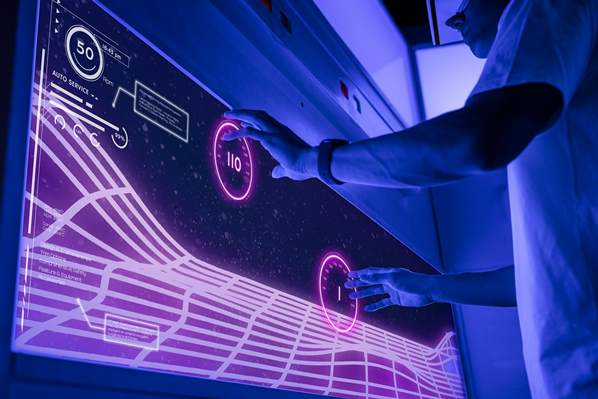
Managing project resources, especially in a multi-project environment, can be really challenging: you can face resource conflicts, difficulties in forecasting future resource needs, large amounts of data, etc.
This is where AI and predictive analytics in particular come into play. Read this article to learn more about predictive analytics and its applications in AI resource management.
How Does Predictive Analytics Work?
Predictive analytics utilizes historical data, current trends, and machine learning algorithms to forecast future outcomes. In project resource management, predictive models can forecast the following things:
- Human resource requirements (the number of employees needed during specific periods for particular projects);
- Material needs (the quantities of materials or products required based on historical usage);
- Technological or infrastructural needs (predicting when systems might require upgrades or where potential bottlenecks in network capacity could arise).
These insights allow managers to anticipate needs and address them proactively. Such an approach reduces inefficiencies and project lead times.
Required Data Sources and Metrics
Accurate forecasting by AI systems becomes possible thanks to analyzing various data sources. Speaking about forecasting project resource needs, we can mention diverse sources:
- historical records indicating how resources like personnel, materials, and finances have been utilized previously;
- operational metrics such as project completion rates, employee productivity, machine utilization, and supply chain timelines;
- seasonal or market trends (external factors like market shifts, customer behavior, or seasonal cycles that influence demand);
- real-time data from IoT devices, sensors, and other systems feed live information to predictive models.
By synthesizing these diverse data points, AI systems can provide you with resource utilization patterns so that you can make reliable predictions for future resource needs.
The Use of Predictive Analytics in Project Resource Management
Let’s now consider the areas where predictive analytics increases the effectiveness of AI resource management.
1. Forecasting resource demand.
Predictive analytics helps project managers forecast future demand for employees, materials, and equipment. AI-driven resource management software can analyze previous projects, workloads, and team members’ performance to estimate resource requirements for future projects. As a result, project/resource managers can plan and allocate resources more effectively, avoid risks of resource shortages and last-minute hiring.
2. Resource allocation.
Predictive analytics can assess employees’ competences, availability, capacity, or other attributes to assign the right people to the right tasks. This helps utilize resources efficiently, prevent them from improper workloads, and facilitate team members’ productivity.
3. Risk mitigation.
With resource management software equipped with predictive analytics capabilities, you can identify potential risks related to resources or the project flow, e.g., resource shortage, equipment failure, project delays, etc. Detecting these risks in advance helps project or resource managers take timely measures to prevent or mitigate them: reallocate resources, adjust milestones, postpone other projects, etc. With this approach, the possibility of disruptions in the project flow is reduced.
4. Capacity planning.
Predictive analytics can anticipate peaks and troughs of resource demand, which helps managers perform effective resource capacity planning. They can forecast when they will need employees with specific skills or when extra people will be required. Informed capacity planning helps prevent resource shortages, maintain balanced workloads, and avoid bottlenecks.
5. Decision-making.
Predictive analytics enables project and resource managers to forecast various project outcomes by simulating potential changes in resources, timelines, or budgets. This capability allows them to make more informed and reasonable decisions, optimize resource utilization, identify and address bottlenecks, and streamline work across projects.
Therefore, predictive analytics helps resource managers utilize available resources with maximum efficiency, prevent and eliminate bottlenecks, and make data-driven decisions.
However, in order to achieve the above-mentioned objectives and make predictive analytics work appropriately, you need to fulfill certain conditions. We’ll consider them in the next section.
Making Predictive Analytics Deliver Optimal Results
Resource management software with predictive analytics capabilities analyzes project data and transforms it into actionable insights that project managers can leverage to enhance project performance. However, to ensure an AI resource management solution delivers maximum value, several key requirements must be met. Let’s explore them.
Tip 1. Collect high-quality data
The success of leveraging predictive analytics depends on the quality of data it utilizes. So, you should make sure that the data you’re working with is accurate and relevant. Otherwise, the predictions and insights you get won’t be accurate. Therefore, it’s a good idea to establish consistent data collection methods. Collect data from past projects, including resource allocation, timelines, and costs, to forecast future requirements accurately. Also, you should regularly feed real-time data into the system to keep predictions relevant and up-to-date.
Tip 2. Select the right resource management tools
You should choose resource management solutions with incorporated predictive analytics capabilities. If you work in a multi-project environment, make sure that the tool is suitable for multi-project resource management. Finally, check the tool’s scalability and capabilities to integrate with other software systems.
An example of a resource management tool with predictive analytics capabilities is Epicflow. It’s designed to meet the needs of a multi-project environment with a shared resource pool. Therefore, its functionality helps achieve resource efficiency, minimize bottlenecks, and optimize work on multiple concurrent projects.
3. Train team members to use tools correctly
Predictive analytics requires human participation. So, you need to instruct your team members to interact with resource management tools effectively. First, they should update the information that requires updates. Secondly, you should explain to them how to interpret the insights provided by predictive analytics and use them in making decisions. Without that, RM tool with predictive analytics will be useless.
4. Use regular real-time monitoring
As a rule, RM tools have dashboards reflecting real-time progress, project and resource performance, resource utilization, and more. To increase the effectiveness of work with predictive analytics resource management solutions, you should regularly monitor all indicators and timely react to any detected issues.
Conclusions
Let’s now draw some conclusions.
- Predictive analytics is a subset of AI, which is widely used in project and resource management to forecast future outcomes.
- Predictive analytics is used in AI resource management in the following areas: demand forecasting, resource allocation, resource capacity planning, risk management, and decision support.
- To make predictive analytics work to your advantage, you should create appropriate conditions: gather high-quality data, choose the right resource management tool, train your team members to gain insights from it, and apply regular monitoring and control.