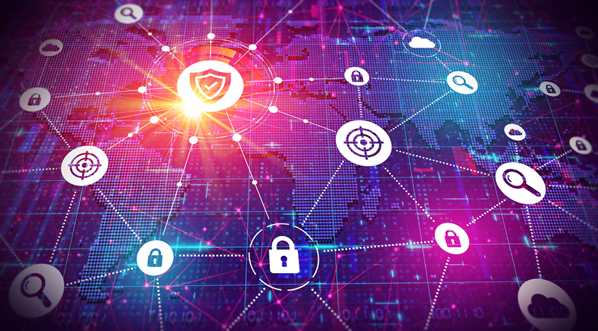
As organizations move towards cloud-based environments and enable remote work, adopting a Zero Trust Security model has become a necessity.
The core principle of Zero Trust is simple yet powerful: never trust, always verify . It assumes that no user, device, or system—whether inside or outside the network—should be trusted by default. Continuous verification and validation are key to ensuring security.
Incorporating Artificial Intelligence (AI) and Machine Learning (ML) into Zero Trust frameworks is a game-changer. These technologies offer the ability to analyze vast amounts of data in real-time, identify anomalies, and automated threat detection, significantly enhancing the effectiveness of Zero Trust models. In this article, we’ll explore how AI and ML play a crucial role in reinforcing Zero Trust Security by improving anomaly detection, automating threat response, and enabling more intelligent decision-making.
Understanding Zero Trust Security
Zero Trust Security is based on the concept that a network should be treated as though it is always potentially compromised. Unlike traditional models that rely on a trusted internal perimeter , Zero Trust operates under the assumption that every user, device, and application must be continuously verified.
The key principles of Zero Trust include:
- Least Privilege Access: Users and devices are only granted the minimal level of access they need to perform their tasks, reducing the attack surface.
- Continuous Authentication: Identity verification is ongoing and not limited to the initial login, ensuring that users are continuously validated as they interact with resources.
- Micro-Segmentation: The network is divided into smaller, isolated segments to prevent lateral movement in the case of a breach.
While Zero Trust can significantly improve security, implementing it requires a thoughtful approach. Businesses need to assess their current infrastructure, integrate Zero Trust policies, and manage user and device access. Learn how to implement Zero Trust Security in your organization to ensure seamless integration and a stronger defense against cyber threats.
AI and Machine Learning in Cybersecurity
Artificial Intelligence and Machine Learning are transforming the way cybersecurity professionals detect, analyze, and respond to threats. These technologies enable the automation of complex tasks, enhance threat detection accuracy, and improve decision-making speed. Here’s how AI and ML contribute to cybersecurity:
- Anomaly Detection: AI algorithms can monitor network activity and detect deviations from established patterns. For example, if an employee accesses a resource they don’t usually interact with, AI can flag this as a potential risk.
- Behavioral Analytics: By analyzing user and device behavior, AI can detect suspicious activities, such as a user accessing sensitive data outside of normal business hours or from an unusual location.
- Predictive Threat Detection: AI can predict potential vulnerabilities based on historical data and ongoing trends, helping organizations proactively protect their systems before an attack occurs.
- Automation of Threat Response: AI can not only detect threats but also trigger automated responses, such as isolating compromised devices or blocking suspicious user accounts, reducing the time it takes to contain an attack.
How AI Enhances Zero Trust Security
Zero Trust Security is built on the idea of continuous validation and least privilege access, but it’s difficult to manually monitor all aspects of a network in real-time. This is where AI and ML come into play. By incorporating AI into Zero Trust models, organizations can automate and streamline many security processes that would otherwise be time-consuming and prone to human error. Here’s how AI enhances Zero Trust Security:
1. Anomaly Detection and Behavioral Analysis
AI excels at detecting deviations from normal behavior. In a Zero Trust framework, this capability is crucial for identifying potential threats early. For example, AI can analyze patterns of user and device behavior and flag any irregular activities that could indicate a compromise.
Consider the example of a legitimate user who normally accesses company data only from the office. If that user suddenly logs in from a different country or tries to access sensitive files they don’t typically interact with, AI would flag these behaviors and trigger an alert, prompting further investigation.
This continuous monitoring and behavioral analysis ensures that only authorized users and devices gain access to critical systems, and any unusual activity is immediately addressed.
2. Real-Time Threat Detection
In a Zero Trust environment, all users and devices are assumed to be untrusted until verified. AI enhances this model by enabling real-time threat detection. Through AI-powered monitoring systems, organizations can assess the security status of users , devices, and applications at any given moment. If an anomaly is detected—such as a sudden spike in network traffic or unauthorized access to a high-value asset—AI can quickly identify the threat and trigger an automatic response, like isolating the device or limiting user access.
AI’s ability to process data at scale and in real-time allows organizations to stay one step ahead of attackers, ensuring that potential threats are mitigated before they escalate.
3. Automating Threat Response
AI’s most significant contribution to Zero Trust Security is the automation of threat responses. Zero Trust requires continuous monitoring and verification, but without AI, this would overwhelm security teams. AI tools can automatically assess threats and decide on the appropriate action, such as:
- Blocking access to a compromised account.
- Alerting security teams to further investigate a suspicious event.
- Automatically isolating infected devices from the network to contain the threat.
This automation reduces the time it takes to react to security incidents, ensuring a faster and more efficient response to attacks.
4. Integration with Zero Trust Policies
AI complements Zero Trust by enforcing security policies dynamically. Traditional security systems rely on predefined rules and static configurations, but AI can adjust security measures based on real-time data. For example, if AI detects that a user is accessing the system from an unrecognized device, it could trigger a policy to require multi-factor authentication (MFA) or limit access to certain resources.
By continuously adapting to changing threats and behaviors, AI ensures that the Zero Trust model is always up to date and capable of protecting against new and evolving risks.
Challenges and Limitations of Using AI in Zero Trust Security
While AI is a powerful tool for enhancing Zero Trust Security, it’s not without challenges:
- False Positives and Negatives: AI systems can occasionally misidentify legitimate activities as threats or fail to detect more subtle attacks. This is especially true in the early stages of AI integration when models are still learning.
- Complexity and Integration: Incorporating AI into an existing Zero Trust framework can be complex, requiring careful planning and execution. AI systems need to be trained on large datasets, and security teams must ensure that they’re fully integrated with other security measures.
- Data Privacy Concerns: Continuous monitoring of user behavior can raise privacy concerns, especially in industries where sensitive personal data is involved. Organizations must balance the need for security with the need to protect user privacy.
To mitigate these challenges, organizations should combine AI with human oversight and ensure that the systems are continuously updated with the latest data and threat intelligence.
The Future of AI in Zero Trust Security
As AI continues to evolve, its role in Zero Trust Security will only become more significant. Some emerging trends include:
- AI-Powered Threat Intelligence: By analyzing vast amounts of global cybersecurity data, AI will help organizations identify emerging threats more quickly.
- Self-Learning AI Systems: AI models will continue to improve their detection capabilities over time, learning from new data and adapting to new types of attacks.
- Quantum Computing and AI: Advances in quantum computing may enable even more powerful AI models, capable of processing data at unprecedented speeds and accuracy.
Human expertise will still play an essential role in overseeing AI systems and ensuring that security policies align with business needs. However, AI will continue to be an indispensable tool in combating cyber threats in a Zero Trust environment.
Conclusion
AI and ML are transforming the way organizations approach cybersecurity, particularly in the context of Zero Trust Security. By automating threat detection, analyzing behavior, and continuously verifying access, AI strengthens Zero Trust frameworks and helps organizations stay ahead of evolving cyber threats. However, businesses must be aware of the challenges AI brings and take a balanced approach that combines technology with human oversight. As AI continues to advance, its role in Zero Trust Security will only become more integral, making it an essential tool for securing modern networks.